Navigating the Promise and Peril of Large Language Models
From art to ethics, Blackbird.AI's Paul Burkard charts the complex landscape of generative AI and large language models.
Posted by Blackbird.AI’s RAV3N Narrative Intelligence and Research Team on February 28, 2024

Few technologies have captured the public’s imagination like large language models (LLMs) – artificial intelligence systems trained on massive amounts of data that can rapidly generate human-like writing, speech, images, and even video.
LLMs like OpenAI’s ChatGPT, Google’s Gemini, Anthorpic’s Claude, and others have demonstrated an impressive ability to produce coherent, thoughtful responses to natural language prompts. They can summarize lengthy documents, compose poetry, answer questions, and generate code. Most systems have been developed responsibly, and their capabilities have improved exponentially thanks to computing power and neural network design advances.
But as LLMs grow more powerful, not all systems have guardrails. AI experts have raised concerns about potential misuse. Paul Burkard, Director of AI at Blackbird.AI, explains the promise and risks posed by generative AI.
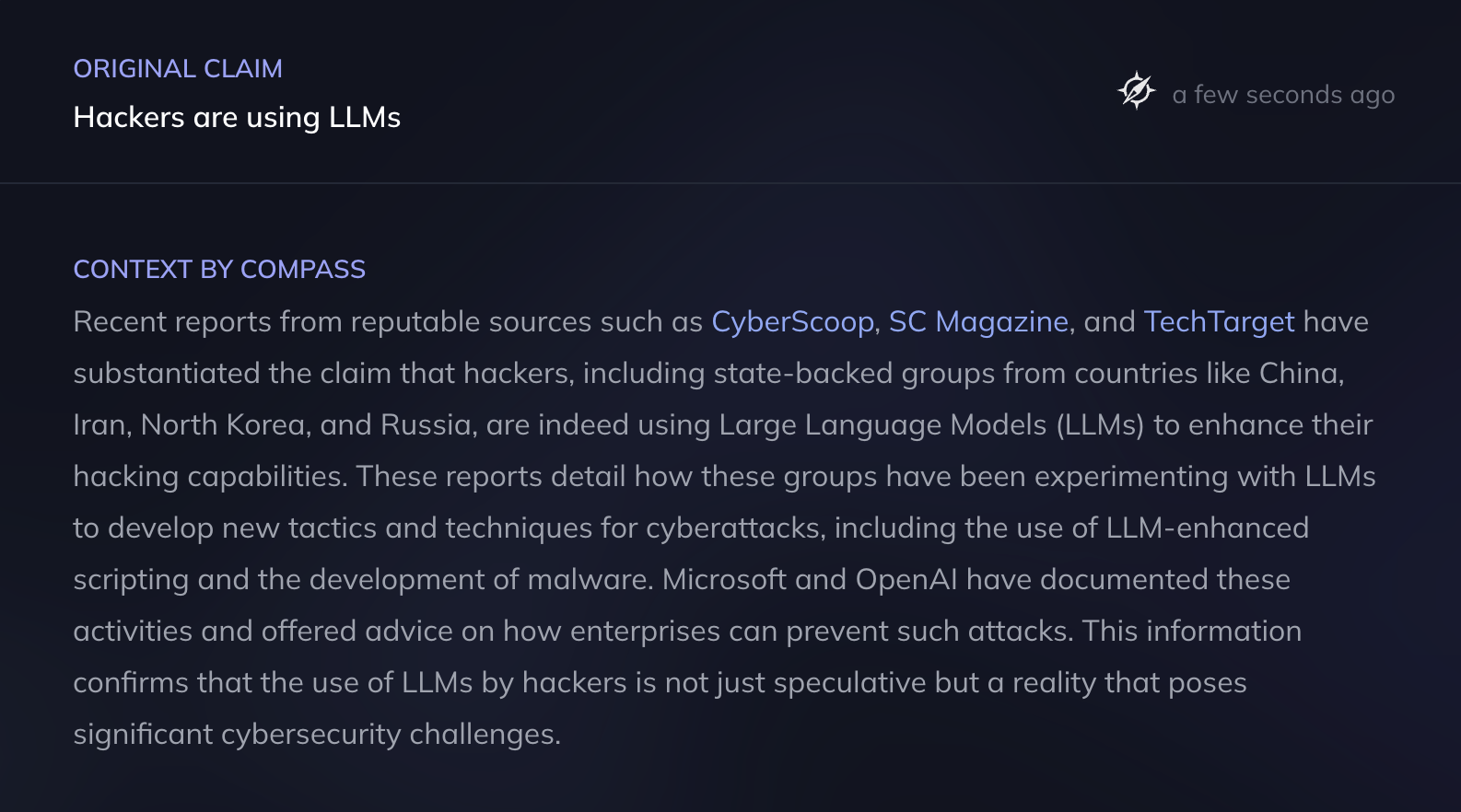
How LLMs Work
LLMs like ChatGPT are a type of foundation model – an AI system trained on broad data that can be adapted to various downstream tasks. Foundation models learn representations of the world from their training data, allowing them to make connections and generate outputs based on patterns they’ve observed.
“It’s a world model that takes in tons and tons of data and learns how to make connections,” Burkard explained. “So when you input something, it will output a probabilistic string of tokens based on everything it’s ever seen.”
Training is key to developing these capabilities. During training, LLMs ingest massive text datasets – on the scale of hundreds of billions of words. The model learns complex language representations as it’s exposed to more examples of natural language use.
Recent advances in reinforcement learning have boosted performance further. “You have human experts give it input. It sends an output. And they rate the output, and it gets better quickly,” said Burkard. This human feedback allows the model to improve and generate more human-like responses rapidly.
Applications and Ethical Concerns
LLMs have demonstrated potential for myriad applications, from speech and language therapy to computer programming. Their ability to summarize lengthy texts could aid knowledge workers and academics. Creative professionals are also experimenting with LLMs to generate ideas and content.
However, the same capabilities that enable beneficial applications also raise concerns about misuse. Generating deceptive political messaging and spam at scale would be trivial for an LLM. The risks include impersonation and fraud through generated audio, video, and text.
“It doesn’t take a lot. If you get behind the curtain and become the new teacher…it doesn’t take a lot to make it unlearn what it was taught for being a safe model,” Burkard said regarding removing constraints from LLMs.
LLMs could supercharge phishing, hacking, and cyber attacks by cheaply generating extremely naturalistic, personalized messaging to targets. According to Burkard, detecting machine-generated fake media will likely become more difficult, while generating it will become easier.
Mitigating Risks
Given the potential for harm, what can mitigate risks while allowing beneficial uses? Regulation will likely require proof of authenticity and human origin for published media.
“A common suggestion is that the content that is machine-generated should be watermarked to indicate that it could be AI-generated,” Burkard explained. “This is similar to the long-existing practice of physical watermarking to establish authenticity, but in the digital world. The giants of the generative AI space have all signed on to watermarking pledges, but watermarking is no panacea. Many experts have even expressed doubt that it will be useful at all. Others suggest it could be a part of the solution, alongside potential things like cryptographic techniques to establish provenance better. Cryptographic wars against bad actors are not a new concept either, and they will never stop all bad actors, but the primary goal is harm reduction by thwarting most low-level attacks.”
But perfectly distinguishing real from fake media may only be feasible in the short term. Ultimately, the solution may be societal adaptation and education. Burkard noted that younger generations innately understand online risks better, having grown up with digital technology. Targeted education programs could help the public identify and adapt to synthetic media risks.
The Way Forward
The full implications of LLMs still need to be clarified. How might they impact geopolitics in the coming years? Burkard pointed to the 2024 U.S. presidential election as a potential inflection point. With one candidate more inclined towards no-holds-barred tactics, there are concerns about computer-generated disinformation overwhelming the public discourse.
The digital security landscape is set to become increasingly treacherous as cybercriminals and other bad actors employ a mix of automated tools, artificial intelligence, and complex social engineering techniques. But by implementing AI-driven narrative intelligence solutions now, revising policies, and educating decision-makers, we can identify and counteract both the technical and narrative-based elements of weaponized LLMs. Despite the continuous evolution of threat actors, being well-informed and gaining insights into these attacks remains our strongest defense to make better strategic decisions when a crisis hits.
The arms race between beneficial and harmful applications of LLMs will also continue. OpenAI recently unveiled a tool for detecting AI-written text, but many believe LLMs will soon get good enough to evade detection. It’s impossible to predict where exactly the technology will go from here.
As technology advances, so too do narrative threats enabled by artificial intelligence. Bad actors can leverage LLMs to carry out narrative attacks effectively. Ultimately, while the online landscape grows more hazardous, our best defense comes from comprehending the narratives weaponized against us and responding decisively. Tools like Compass by Blackbird.AI can provide crucial buttress by providing context to misinformation and disinformation.
LLMs provide a glimpse of the creative potential of AI while posing complex ethical dilemmas. Developing governance and norms to prevent misuse is now an urgent priority. Education can help society adapt. Ultimately, we must thoughtfully navigate the promises and risks as this technology advances rapidly. The AI genie is out of the bottle – now we must figure out how to responsibly coexist.
To learn more about how Blackbird.AI can help you in these situations, contact us here.
About Blackbird.AI
BLACKBIRD.AI protects organizations from narrative attacks created by misinformation and disinformation that cause financial and reputational harm. Powered by our AI-driven proprietary technology, including the Constellation narrative intelligence platform, RAV3N Risk LMM, Narrative Feed, and our RAV3N Narrative Intelligence and Research Team, Blackbird.AI provides a disruptive shift in how organizations can protect themselves from what the World Economic Forum called the #1 global risk in 2024.